Research Program
Our research program sits at the intersection of evolutionary ecology and computational systems, with a particular focus on developing foundation models for organismal biology. We develop novel AI architectures informed by biological theory to understand complex eco-evolutionary systems.
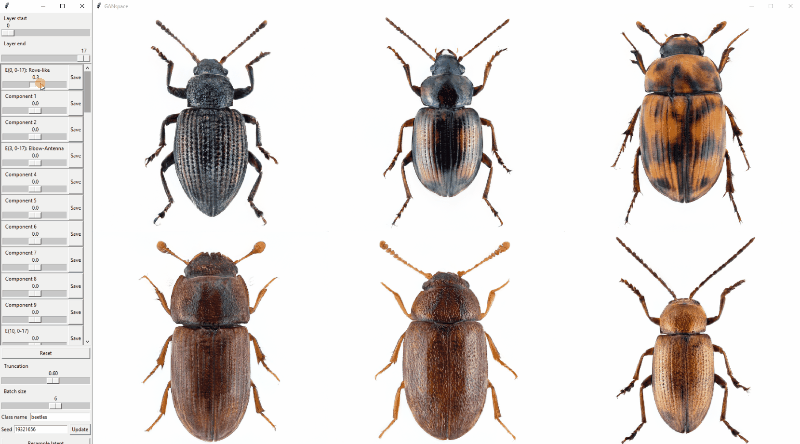
Research Vision: Bridging Computation and Natural Systems
Foundation models have transformed fields from language processing to protein folding, yet their potential in organismal biology remains largely untapped. Our research addresses this gap by developing novel AI architectures informed by biological theory. Rather than simply applying existing AI tools, we create new approaches that integrate biological principles - like phylogenetic relationships and ecological constraints - into their fundamental design.
The theoretical foundation of this work rests on a key insight: biological systems exhibit complex, high-dimensional structure that can be effectively captured through modern deep learning approaches, particularly when those approaches are constrained by biological first principles.
Current Research Directions
1. Foundation Models for Biological Understanding
Our NSF-funded research is developing NicheFlow, one of the first true foundation models for ecology. This generative AI system learns the underlying structure of species' environmental niches, enabling accurate predictions even for rare or data-deficient species. NicheFlow demonstrates how AI can help solve critical conservation challenges by providing a unified framework for predicting species distributions with minimal data, generating hypothetical niches to explore potential responses to climate change, and creating accessible tools for conservation planning.
We are committed to developing these models ethically and responsibly, incorporating diverse stakeholder perspectives from the outset. For NicheFlow, we established an advisory board including AI ethicist Clinton Castro to ensure the model's development considers potential risks and biases. The project emphasizes open science principles, with model development happening transparently and collaboratively.
2. High-Throughput Phenomics and Digital Specimens
Through collaboration with the NSF-funded Phenobase project, we helped develop PhenoVision, an AI framework that achieves exceptional accuracy (98.5% for flowers, 95% for fruits) in detecting reproductive structures from field photographs while processing over 53 million images. This system addresses critical data gaps in global phenology monitoring, particularly in understudied regions, adding information for over 119,000 species across 10,400 genera.
This experience demonstrated how to successfully operationalize AI models "in the wild." Building on this experience, we are developing new approaches to digital phenotyping that combine AI-enabled analysis with novel data collection strategies. This includes frameworks for "digital-first specimens" - comprehensive digital representations that capture more information than traditional preservation methods while minimizing environmental impact.
3. Evolution in High Dimensions
Our research investigates how AI systems themselves can serve as model organisms for understanding evolutionary processes. The theoretical foundation for this work comes from recent advances in manifold learning and geometric deep learning, which have revealed that many complex, high-dimensional systems actually evolve on lower-dimensional manifolds constrained by underlying physical or biological principles.
By studying how different training histories affect model performance - for instance, how pre-training on species identification improves flower detection capability - we gain insights into how constraints and contingencies shape adaptation. These artificial systems provide unprecedented opportunities to study evolution in high-dimensional spaces, complementing traditional theoretical approaches that often rely on low-dimensional approximations.
This work has revealed striking parallels between the training dynamics of deep learning models and biological evolution. Just as organisms evolve through a combination of variation, selection, and inheritance, our models learn through analogous processes of parameter perturbation, loss minimization, and weight updates.
We have applied some of the ideas already to generative model of 3d bird beak shape, based on museum specimen scans, bridging between this theme and our high-throughput phenomics theme.
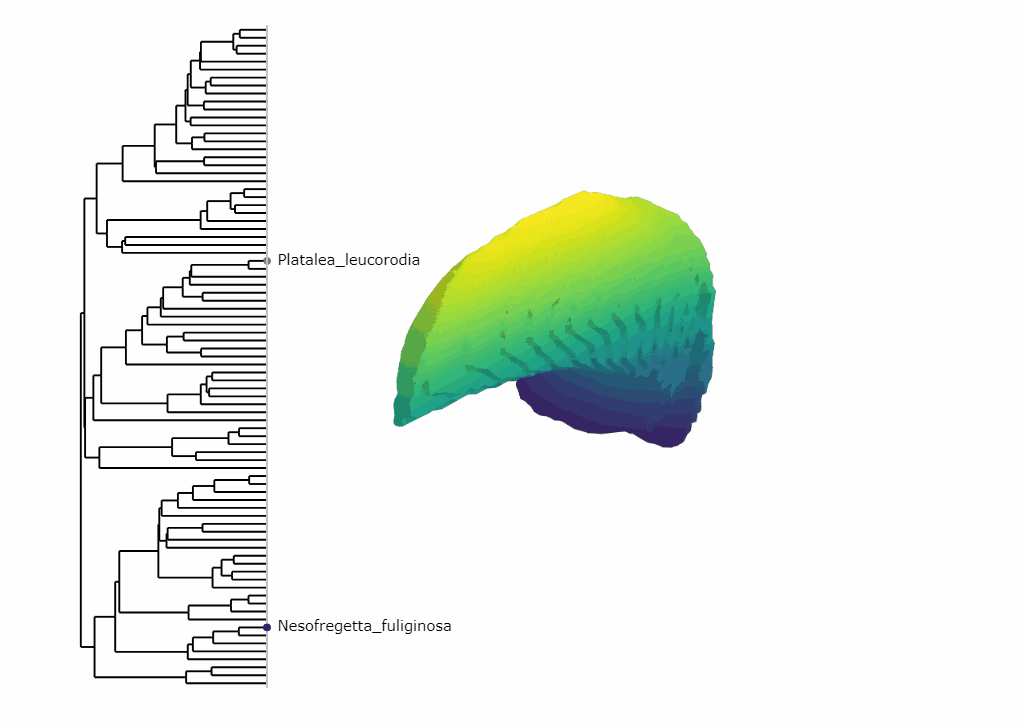
Future Research Directions
New Frameworks for Biological Inference
A major direction of our research program is developing novel foundation models for inferring biological processes from complex data. Building on recent advances in Prior-Data Fitted Networks (PFNs) and leveraging our experience developing complex simulation frameworks, we are creating systems that can learn implicit prior distributions over complex data-generating processes through simulation-based training.
This framework will enable estimation of posterior distributions over process parameters directly from complex, high-dimensional data - whether that's 3D morphological scans, hyperspectral imagery, or genomic sequences. By training on simulated data generated from mechanistic models, these networks learn to recognize signatures of different biological processes, making sophisticated Bayesian inference accessible to researchers across biological subdisciplines.
Expanding High-Throughput Phenomics
Working with museum collaborators at leading institutions, we are developing new approaches to high-throughput digital phenotyping that go beyond simple measurement. By combining advanced computer vision with geometric deep learning techniques, we create models that learn meaningful representations of organismal form directly from raw 3D scans or multi-spectral images.
Understanding Evolution in Complex Systems
Our ongoing work investigating AI systems as models for evolutionary processes is expanding to study how different pre-training strategies and architectural constraints influence the development of complex capabilities. This research direction maintains strong potential for traditional scientific funding while developing tools with broad applications in both basic research and practical implementation.
Collaborative Vision
Our research program thrives through strategic collaborations that bring together diverse expertise. By working with museum curators, field biologists, computational scientists, and conservation practitioners, we create integrative approaches that bridge theoretical advances with practical applications. These partnerships enable access to exceptional resources including extensive biological collections, advanced computational infrastructure, and field sites for testing and validating models.
Drawing on our experience with PhenoVision, we lead initiatives that bridge the gap between model development and practical implementation. This includes establishing interdisciplinary teams that bring together domain experts, end users, and AI specialists to ensure models achieve their intended impact.
Cross-Cutting Themes
Our past and future work falls into several cross-cutting themes that drive our research program:
Phylogenetic and Spatial Comparative Methods
How species' traits are determined by their evolutionary history and contemporary interactions. Space influences traits in ways with many similarities, so I also work on combining them together.
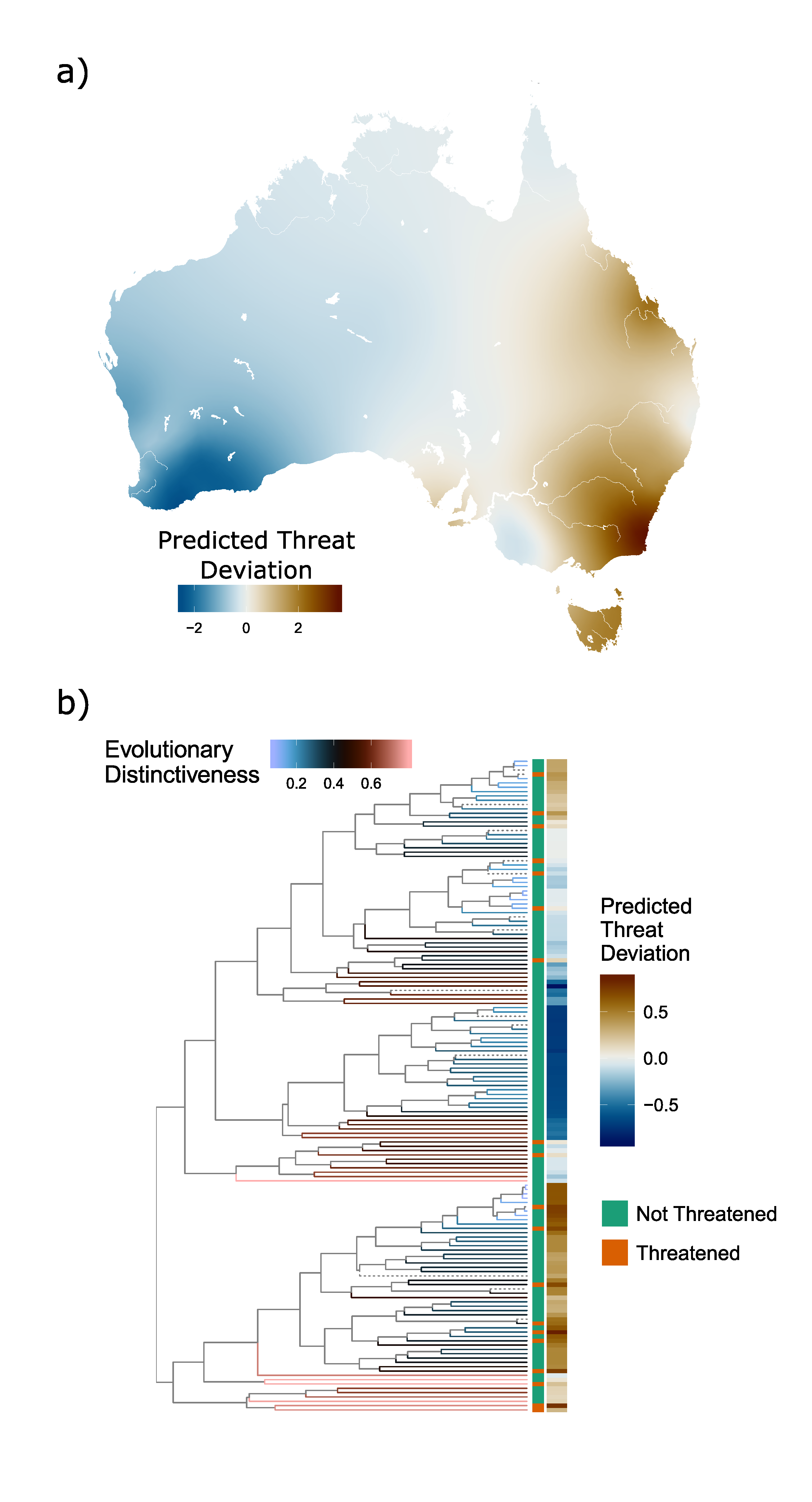
Phylogenetic Community Ecology
How long-term evolutionary processes constrain and shape the interactions of coexisting communities of species.
Species Distribution Modelling
How species' biology determines their interaction with the environment and each other, while accounting for their evolutionary connections.
Cultural Comparative Analysis
How evolutionary connections between human cultures can be used when asking contemporary questions about human behavior and culture.
Simulation and Theoretical EEB
Understanding the processes involved in eco-evolutionary systems and their long-term outcomes and consequences.